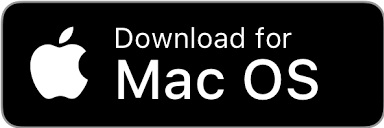


We will perform the analysis through the Solver command. Now, we will conduct the final regression analysis. Step 6: Use Solver Analysis Tool for Final Analysis
#LOGISTIC REGRESSION FLATICON HOW TO#
If you don’t know how to input the Absolute Cell Reference sign, you can input it in several ways. Use the Absolute sign to freeze the cell value of variables. Write down the following formula in cell E5.Here, b0, b1, and b2 are regression variables. We define the Logit value as X in our calculation. In this step, we are going to calculate the Logit value for our dataset. Read More: Multiple Linear Regression on Excel Data Sets (2 Methods) We are assuming all the variables’ values as 0.01.Then, input your Solver Decision Varibles’ We input them in the range of cells D16:D18.For our analysis, we input the dataset in the range of cells B5:D14. First of all, input your dataset accurately into Excel.In this step, we are going to import your dataset: The whole analysis procedure is explained below step by step: The values initial regression solver variable are in the range of cells C16:D18. So, our dataset is in the range of cells B5:D14. The age of those machines is in column C and the average duty hours of them per week is in column D. The binary digits 1=positive, and 0=negative, and these values are shown in column B. The machine’s availability can be positive or negative. To perform the analysis, we consider a dataset of 10 machines from an industry. This type of analysis provides us with a prediction value of the desired variable. In this article, we will perform the binary logistical regression analysis. Step-by-Step Procedure to Do Logistic Regression in Excel However, in this model, we need a predetermined order to categorize them. Ordinal Logistic Regression: This regression analysis model works for more than two categories. If we have more than two classified sections to categorize our data, then we can use this regression analysis model. Multinomial Logistic Regression: Multinominal logistic analysis works with three or more classifications. Logistic regression analysis mainly three types:īinary Logistic Regression: In the binary regression analysis model, we define a category by only two cases. It helps a person to get the result from a large dataset based on his desired category. In the case of a Precision-Recall tradeoff, we use the following arguments to decide upon the threshold:-ġ.Logistic regression analysis is a statistical learning algorithm that uses to predict the value of a dependent variable based on some independent criteria. Ideally, we want both precision and recall to be 1, but this seldom is the case. The decision for the value of the threshold value is majorly affected by the values of precision and recall. The setting of the threshold value is a very important aspect of Logistic regression and is dependent on the classification problem itself. Logistic regression becomes a classification technique only when a decision threshold is brought into the picture. Just like Linear regression assumes that the data follows a linear function, Logistic regression models the data using the sigmoid function. The model builds a regression model to predict the probability that a given data entry belongs to the category numbered as “1”. In a classification problem, the target variable(or output), y, can take only discrete values for a given set of features(or inputs), X.Ĭontrary to popular belief, logistic regression is a regression model. Logistic regression is basically a supervised classification algorithm. This article discusses the basics of Logistic Regression and its implementation in Python. Decision Tree Introduction with example.Removing stop words with NLTK in Python.ML | Momentum-based Gradient Optimizer introduction.Optimization techniques for Gradient Descent.ML | Mini-Batch Gradient Descent with Python.Difference between Batch Gradient Descent and Stochastic Gradient Descent.Difference between Gradient descent and Normal equation.ML | Normal Equation in Linear Regression.Mathematical explanation for Linear Regression working.Linear Regression (Python Implementation).

Regression and Classification | Supervised Machine Learning.Basic Concept of Classification (Data Mining).Gradient Descent algorithm and its variants.ISRO CS Syllabus for Scientist/Engineer Exam.

ISRO CS Original Papers and Official Keys.GATE CS Original Papers and Official Keys.
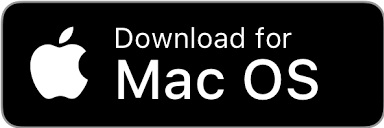